Plant Metabolic Networks and their Organization
The first characteristic feature of plant metabolism is its biosynthetic capacity
(Croteau
et al.,2000; Wink, 1999).While bacterial and yeast metabolisms encompass
only a few hundred metabolites, the number of known plant secondary products is
estimated to be 100,000 (Schwab, 2003), and the actual number may be as high as 200,000 (Sumner
et al., 2003). Obviously individual species synthesize only a particular
subset of these compounds, but any attempt to define the metabolic network in
a plant cell has to include substantially more biosynthetic pathways than in a typical
microorganism. Moreover, since the manipulation of the fluxes through these pathways
can be of agronomic and commercial interest (Dixon and Sumner, 2003), the
definition of the secondary pathways in the metabolic network may be as important
as the definition of the pathways of central metabolism in generating predictive
models appropriate for metabolic engineering.
Another characteristic and well-known feature of plant metabolism is the
extensive subcellular compartmentation that occurs within a typical plant cell
(ap Rees, 1987). The cytosolic, plastidic, peroxisomal, and mitochondrial compartments
are all metabolically important, with the plastids in both heterotrophic
and photosynthetic cells having a notable role in biosynthesis. In some cases,
particular metabolic steps occur uniquely in one compartment, for example, the
synthesis of starch from ADPglucose is exclusively plastidic, but there are many
instances where a particular step occurs in more than one compartment, and in
extreme cases this leads to the duplication of whole pathways in two or more
compartments. For example, there is considerable duplication of the pathways of
carbohydrate oxidation between the cytosol and the plastids of heterotrophic
tissues (Neuhaus and Emes, 2000) and many of the reactions of folate-mediated
one carbon metabolism can occur in three compartments—the cytosol, mitochondria,
and plastids (Hanson
et al., 2000). Subcellular compartmentation has two
major consequences for defining the metabolic network and constructing a predictive
model of plant metabolism, and these are discussed in the following
paragraphs.
First, it is necessary to identify all the transport steps that link the subcellular
metabolite pools as well as the subcellular location(s) of each metabolic step. New
plastidic transporters are still being identified (Weber
et al., 2005), and when
added to the multiple metabolite transporters in the inner mitochondrial membrane
(Picault
et al.,2004), the result is to add considerably to the complexity of the
plant metabolic network. Moreover, identifying the subcellular location(s) of
particular steps can be difficult because of the uncertainties associated with the
preparation of sufficiently pure subcellular fractions from tissue extracts, and the
result in any case is often both species and tissue specific. For example, the extent
to which all the enzymes of the pentose phosphate pathway are present in the
cytosol is variable (Debnam and Emes, 1999; Kruger and von Schaewen, 2003),
and our understanding of the pathway of starch synthesis in cereal endosperm
has had to be revised following the characterization of a cytosolic isoform of the
normally plastidic ADPglucose pyrophosphorylase (Burton
et al., 2002; Denyer
et al., 1996).
Second, identical steps in different compartments are generally catalyzed by
isozymes with distinct properties. Thus, duplication of pathways complicates the
construction of predictive models by increasing the amount of kinetic and regulatory
information that is required for the network. Moreover, the subcellular
concentrations of substrates, coenzymes, and effectors will usually be different in
different compartments (Farré
et al., 2001), increasing the information that is required for the construction of a realistic model. A further complication is that
even when an activity has been localized to a compartment, it may be distributed
nonuniformly and in this situation there is the possibility that the effective concentrations
of the substrates, coenzymes, and effectors will differ from their overall
values. Thus, in the case of several cytosolic enzymes, there is good evidence for a
membrane-associated subfraction that can be expected to have distinct kinetic
properties and presumably a specific functional role within the network. Examples
include nitrate reductase (Lo Piero
et al., 2003; Wienkoop
et al., 1999) and sucrose
synthase (Amor
et al., 1995; Komina
et al.,2002), both of which have forms associated
with the plasma membrane, and the recent demonstration of an extensive
association of the enzymes of glycolysis with the outer mitochondrial membrane in
Arabidopsis (Giegé
et al., 2003).
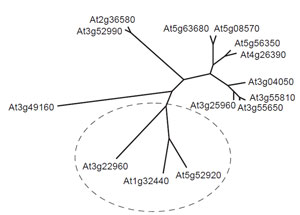 |
FIGURE 1.1 Unrooted phylogenetic analysis of
putative pyruvate kinase genes from Arabidopsis
thaliana. Each gene is identified by its AGI gene
code. The deduced amino acid sequences of
predicted pyruvate kinase isoforms were
compared using CLUSTAL W. Genes proposed
to encode
plastid isoforms of the enzyme were
identified using ChloroP and are enclosed within
the broken
ellipse. Predicted transit peptides
were removed prior to sequence comparison. |
Another important feature of the plant metabolic network is that much remains
to be discovered before a definitive map can be drawn. This assertion is supported
by the discovery of several major pathways in recent years, for example, the pathway
for the synthesis of ascorbate (Smirnoff
et al., 2001) and the methylerythritol
pathway for the synthesis of terpenes (Eisenreich
et al., 2001), and even apparently
well-characterized areas of the network, such as the pathway to ADPglucose in
leaves, can become candidates for reevaluation in the light of new data (Baroja-
Fernandez
et al., 2004, 2005; Munoz
et al., 2005; Neuhaus
et al., 2005). Moreover, the
introduction of new techniques for probing plant metabolism invariably provides
new information about the architecture and regulation of the plant metabolic
network. For example, the development of insertional mutagenesis for gene silencing
has generated a powerful method for probing the redundancy of the network,
and this technique has been used to investigate the interaction between peroxisomes
and mitochondria in plant lipid metabolism(Thorneycroft
et al., 2001). There is also a
very strong indication from the
Arabidopsis and rice genomes that much remains to
be identified before a complete metabolic network can be constructed.
It is already
apparent from the incompletely annotated genomes that many of the identified
enzymes exist in multiple isoforms, and a notable example of this phenomenon is
provided by pyruvate kinase, which appears to be represented by up to 14 genes in
Arabidopsis (Fig. 1.1). Presumably different isoforms play significant roles in particular
compartments of particular cell types at appropriate stages in the plant life cycle,
and incorporating this level of detail into a predictive metabolic model is likely to be
a major challenge.
While the complexity of the plant metabolic network is an obstacle to predictive
modeling, it is also a fundamental characteristic of plant metabolism and it
would be unrealistic to imagine that it can be ignored. An analysis of the metabolic
network in
Escherichia coli suggests that increased complexity is a desirable property
for cells exposed to uncontrollable external conditions, conferring robustness
and the ability to function at near optimal rates over a range of physiological
conditions (Stelling
et al., 2002).
This fundamental property of complex systems
undermines the central objective of attempting to manipulate the performance of
the network through genetic engineering, and it emphasizes the importance of
establishing as complete a description of the network as possible. Fortunately,
annotation of the
Arabidopsis and other plant genomes should provide a complete inventory of the catalytic components of various plant metabolic networks in due
course, and while this will not lead to the immediate clarification of the complex
relationships that determine the way in which the enzymes function in such
networks, it will at least define the scale of the problem.
Assuming that the enzymes and their locations can be identified, there is still
much that needs to be determined to define the metabolic network at a level that
is suitable for predictive modeling of fluxes. In particular, as well as defining the
levels of the enzymes and their substrates, it is also necessary to identify all
the regulatory mechanisms that operate in the network. At one level, this requires
the characterization of all the molecular crosstalk that allows the components
of the system to influence enzyme activity through effector-binding interactions;
and at a higher level, and particularly in a system that will generally not be
maintained in a steady state, it is also necessary to define the relationship between
gene expression and the performance of the network, for example, to include the
effects of circadian rhythms, light–dark transitions, and developmental triggers
on enzyme levels. Clearly, the information required to define a metabolic network
at this level of precision is not available for the cells of an organism as complicated
as a higher plant, and indeed it is arguable that the emerging discipline of systems
biology is unlikely to provide it, since the methodological focus is analytical,
concentrating on genome-scale datasets for transcripts, proteins, and metabolites
rather than mechanistic (Sweetlove
et al., 2003).
It is also interesting to note that
transcriptomic and proteomic analysis of simpler systems has not revealed direct
quantitative correlations with metabolic fluxes (Oh and Liao, 2000; Oh
et al>., 2002;
ter Kuile and Westerhoff, 2001), demonstrating that high-throughput methods
are not yet able to provide an effective alternative to the detailed kinetic and regulatory characterization of a metabolic network if the aim is predictive
metabolic engineering.
While this section has emphasized the importance and difficulty of defining a
complete plant metabolic network, the analysis of even an incompletely specified
metabolic network can be informative. For example, genome-scale models of
metabolism have been developed that allow reliable predictions of the growth
potential of mutant phenotypes in
E. coli, even though the analysis is based on
genome annotation that is only 60–70% complete (Edwards and Palsson, 2000a;
Edwards
et al., 2001; Price
et al., 2003). Similarly, a metabolic flux analysis of the
principal pathways of carbon metabolism in
Corynebacterium glutamicum was
sufficiently detailed to identify a substantial diversion of resources into a cyclic
flux involving the anaplerotic pathways (Petersen
et al., 2000).
This observation
provided the basis for a rational manipulation of the system and indeed the
production of a strain lacking phosphoenolpyruvate (PEP) carboxykinase had
the desired effect of decreasing metabolic cycling and increasing lysine production
(Petersen
et al., 2001). Thus, while it is always possible that an incomplete
metabolic model lacks the key feature that determines a relevant property of the
system, worthwhile predictions of metabolic performance can often be made with
such models. Moreover, even incorrect predictions are useful because they may
suggest ways in which the model can be improved.